
HIV
HIV screening programs represent a major tool towards preventing the spread of HIV by promoting regular testing and by encouraging creative prevention methods and messaging. Identifying individuals at high-risk for contracting HIV enables a more targeted and cost-effective approach for prevention programs. To date, however, traditional universal screening programs are costly, labor intensive and don’t always prove to work with identifying individuals at high risk to HIV.
In our approach, we developed a machine learning model using the statistical probability of risk factors as the baseline traits of a patient in a particular geography and the actual result of an HIV test as the outcome, allowing for an advanced supervised learning model to be implemented.
26 questions and a total of 144 variables were collected from 1032 patients in an anonymous manner while providing HIV screening in collaboration with WITS RHI. A primary neural network with Age, Education Level, Gender, Date of Last HIV Test, and Race as variables, was trained using ELISA HIV test results from each patient as ground truth.
This resulted in a neural network with a 0.829 AUC, 0.740 accuracy, and 0.630 precision trained over 160 training cycles. This implies we can with a single primary classifier predict a patient’s HIV status with a 74% accuracy which we expect can be improved by the addition of additional classifiers based on patient sexual behavior and Bayesian priors. The graph shown illustrates the accuracy of range of classifier approaches being evaluated.

Computing the Risk of contracting HIV has many potential use cases, for example improving the impact of current HIVSS programs.
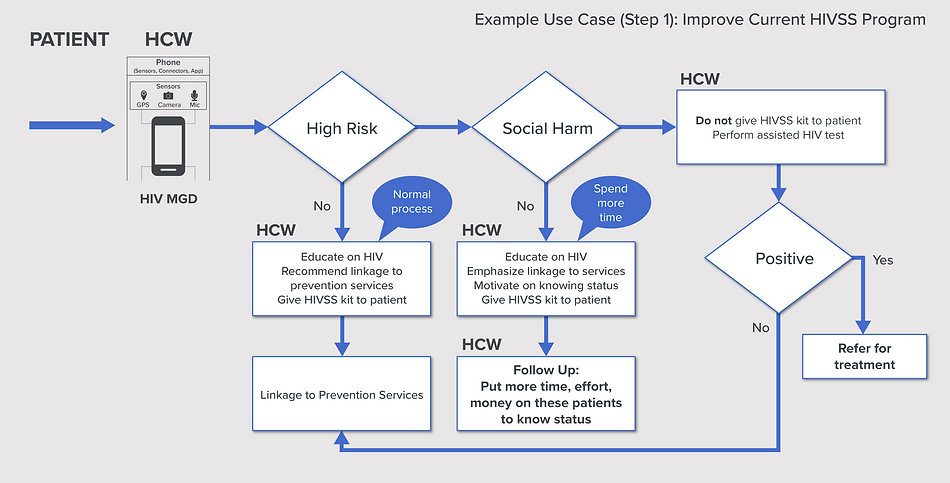
Computing the Risk of contracting HIV can impact positively health metrics such as:
-
Improves the effectiveness of Universal Testing & Treatment - In protocols for HIV eradication that depend on Universal testing every year and treatment, then HIV risk assessment can direct limited testing/treatment resources to the highest-risk groups
-
Increase in number of people knowing their HIV Status
-
Increase in number of people linked to services
-
Reduced “cost to follow-up”
-
Reduced “cost of decision making”
-
Reduced likelihood of duplicate first-time test, which reduces the cost of knowing HIV status
-
Reduction in number of suicides / self-harm cases by identifying self-harm candidates upfront and preventing them from self-screening
-
Support in decision making for putting a person on HIV PREP medication