
AI and LLMs
Developing & implementing AI-based solutions
is a core IPRD capability
IPRD’s team has decades of experience developing computer-vision based solutions for easy-to-use biometric matching, automatic rapid diagnostic test reading, and many other AI-based solutions.
Dr. Keith Hanna, IPRD’s CEO, is a pioneer in innovating and deploying AI-based solutions at scale. IPRD’s advisors also include Dr. Padmanabhan “P” Anandan, founder of WadhwaniAI and Microsoft Research India, and IPRD also works closely with leading research groups, such as Google Research.
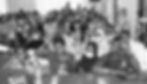
Recent advances in large language models (LLMs) can potentially enable disruptive impact across multiple use cases from health to agriculture in LMICs. IPRD’s extensive experience in research, development, and deployment of advanced AI-based solutions at scale in real-world environments, makes it uniquely positioned to take an LLM use case and turn it into impact.

IPRD Solutions' Dr. Keith Hanna and his team, along with IPRD Advisor Dr. Padmanabhan "P” Anandan, have written a white paper exploring how LLMs can help enhance the lives of poor and underserved communities in Low-and-Middle-Income countries (LMICs). IPRD remains at the forefront of working to understanding how LLMs can fill critical gaps in domains where there are insufficient numbers of trained experts to provide support and care to vast populations.
IPRD’s LLM STRATEGY HAS 3 COMPONENTS: CONTENT, IMPACT and CONTROL
CONTENT
We believe that, since LLMs have largely been trained on information derived primarily from outside LMICs, then our focus on leveraging context-based content, such as WHO Guidelines, local Ministry of Health or Agriculture guidance documents, as well as a patient or other specific record, as examples, will be critical in the effective use of LLMs in LMICs.
LLMs are particularly powerful at summarizing spoken and/or textual content into succinct, actionable steps, for example between a worker and a person in a clinic or community setting.
The approach leverages three collections of information:
-
The patient record (new and old) as normally entered by a HCW into ImpactHealth
-
A WHO ANC guideline document
-
Contextual information on who the participants are in the dialog created by the LLM
Below shows an early example of an LLM capability (based on Chat-GPT4) integrated with IPRD’s ImpactHealth, a Gates-funded program that is resulting in scalable SW capabilities being adopted by Google and WHO (e.g. https://developers.google.com/open-health-stack/stories/iprd).
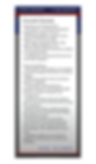
This video demonstrates of a more complete workflow.
IMPACT
While technical assessments can predict the capability of an LLM-based technology, it is much harder to predict whether the capability will have any impact in a particular use case. This is because LLMs involve not just technology but also communication between workers and subjects which includes cultural and workflow limitations and opportunities, and these dynamics can be difficult to predict.
IPRD’s CIM methodology is crucial in this case since these cultural and workflow limitations and opportunities can be rapidly evaluated in the field and rejected or enhanced based on findings measured in real-time.
For example, our early research from the field indicates that task-sharing could be an important disruptive use case for LLM-based technologies. Task-sharing enables co-workers or volunteers who are already in the same environment, with the same subjects or patients, and who already have similar but slightly different specializations or roles, to take over each other's tasks temporarily with the aid of LLM-based technology. This could dramatically multiply the effective workforce in LMICs, allowing different strategies to be used for disease surveillance for example.
In another example, using LLM technology to convey important data within rhyming messages could disruptively appeal to the vast numbers of women who still reach out to traditional practitioners for ANC care. A small example that combines the patient record with a call-to-action for a repeat clinic visit is below.
